How Does Predictive Analytics Transform Esports Betting?
Key Takeaways:
- Predictive analytics combines machine learning and historical data to generate accurate betting predictions
- Real-time match analysis enhances betting accuracy through automated data processing
- AI-driven systems have revolutionized odds calculation and risk assessment
Predictive analytics transforms esports betting by leveraging machine learning algorithms and comprehensive data analysis to create sophisticated forecasting systems. Unlike traditional sports betting that relied on human intuition, modern esports wagering utilizes artificial intelligence to process millions of data points and generate precise predictions.
Data Points That Drive Predictions
- Tournament history and outcomes
- Individual player statistics
- Team performance metrics
- Real-time match information
- Meta-game trends and patterns
How AI Enhances Betting Accuracy
Modern predictive systems analyze multiple factors simultaneously:
- Player performance consistency
- Team composition effectiveness
- Historical match-up results
- Current form indicators
- Environmental variables
The Technology Behind the Predictions
The betting ecosystem employs:
- Machine learning algorithms
- Real-time data processing
- Pattern recognition systems
- Statistical modeling tools
- Automated risk assessment
This technological integration has created a more precise betting environment where data-driven insights guide both bookmakers and bettors. The system continuously learns and adapts, improving prediction accuracy while identifying new patterns and trends in competitive gaming.
Understanding Data Sources in Esports
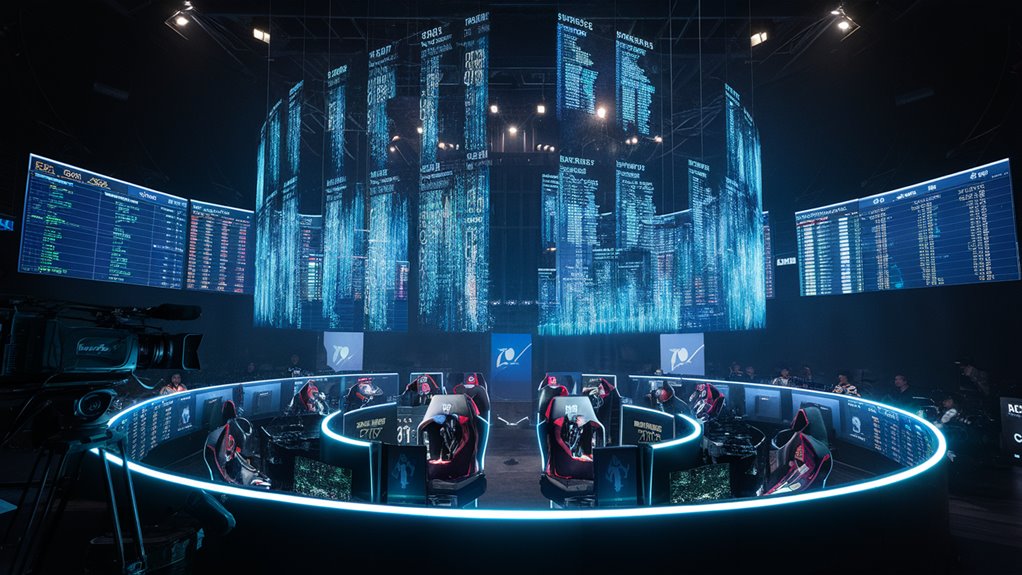
What Are the Key Data Sources for Esports Analysis?
Key Takeaways
- In-game statistics provide detailed performance metrics for predictive modeling
- Player performance tracking reveals individual strengths and competitive patterns
- Historical match data enables comprehensive pattern recognition and trend analysis
Understanding In-Game Statistics
In-game statistics form the foundation of esports data analysis, offering granular insights into player and team performance.
These statistics track essential metrics like kill/death ratios, resource management efficiency, and map control percentages. For analysts and teams, these numbers serve as building blocks for creating accurate predictive models that forecast match outcomes.
Player Performance Metrics
Individual player metrics reveal deeper patterns in competitive gameplay through measurable indicators such as:
- Average Actions per Minute (APM)
- Decision-making patterns
- Role-specific performance indicators
- Consistency levels across tournaments
- Adaptation to different game conditions
Historical Match Analysis
Match history data provides critical context for understanding team and player development over time. Key analysis points include:
- Win rates across different tournaments
- Team composition effectiveness
- Head-to-head performance records
- Impact of patch versions
- Meta changes and adaptations
- Roster adjustment effects
Each match outcome needs evaluation within its full context, considering factors like game updates, team changes, and evolving competitive strategies. This comprehensive approach helps identify reliable patterns and trends that inform future competitive analysis.
Machine Learning Models and Applications
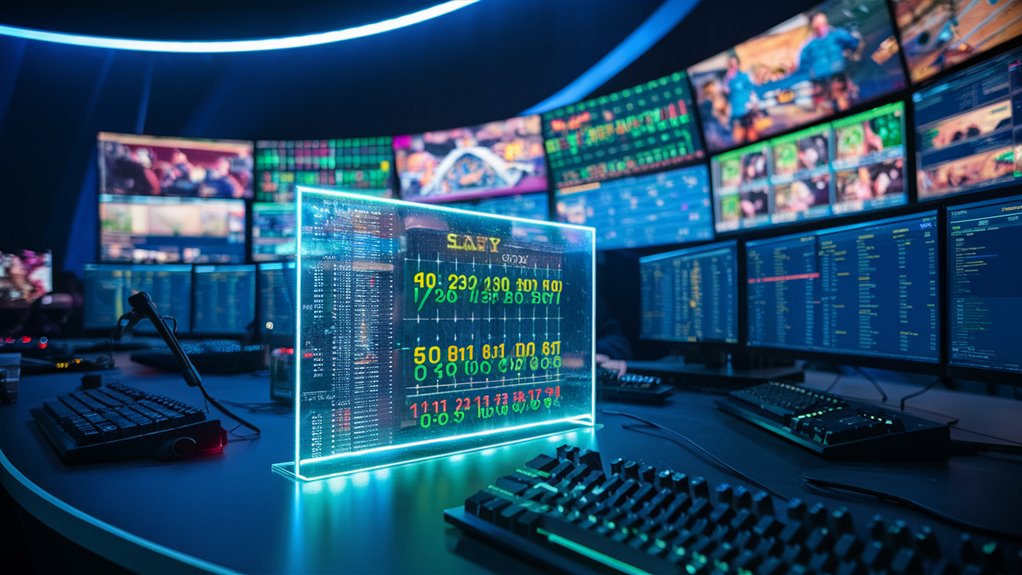
How Do Machine Learning Models Transform Esports Analytics?
Key Takeaways:
- Machine learning algorithms predict match outcomes using historical data and real-time metrics
- Ensemble methods combine multiple specialized models for comprehensive esports analysis
- Neural networks and gradient boosting excel at processing complex gaming patterns
- Real-time data integration enables dynamic predictions and betting optimization
Understanding Predictive Models in Esports
Machine learning transforms esports analytics by combining supervised learning algorithms with comprehensive gaming data to predict competitive outcomes.
Supervised learning algorithms, particularly neural networks and gradient boosting, process historical player performance, team compositions, and meta trends to generate accurate predictions.
Core Applications in Competitive Gaming
Three primary applications drive esports machine learning:
- Win Probability Estimation
- Player Performance Prediction
- Odds Optimization
Random Forest models effectively analyze team dynamics, while Deep Learning networks identify subtle patterns in player behavior and mechanical skill metrics.
Advanced Model Integration
The combination of multiple specialized models creates a robust analytical framework:
- CNNs (Convolutional Neural Networks): Process and analyze gameplay footage
- LSTM Networks: Handle time-series data from match histories
- Real-time Data Processing: Enables dynamic odds adjustments during live matches
These integrated systems continuously adapt to meta changes, maintaining predictive accuracy across different patches and seasons.
Real-time data streams enhance prediction precision, creating more sophisticated in-play betting opportunities and deeper competitive insights.
Real-Time Analytics During Matches
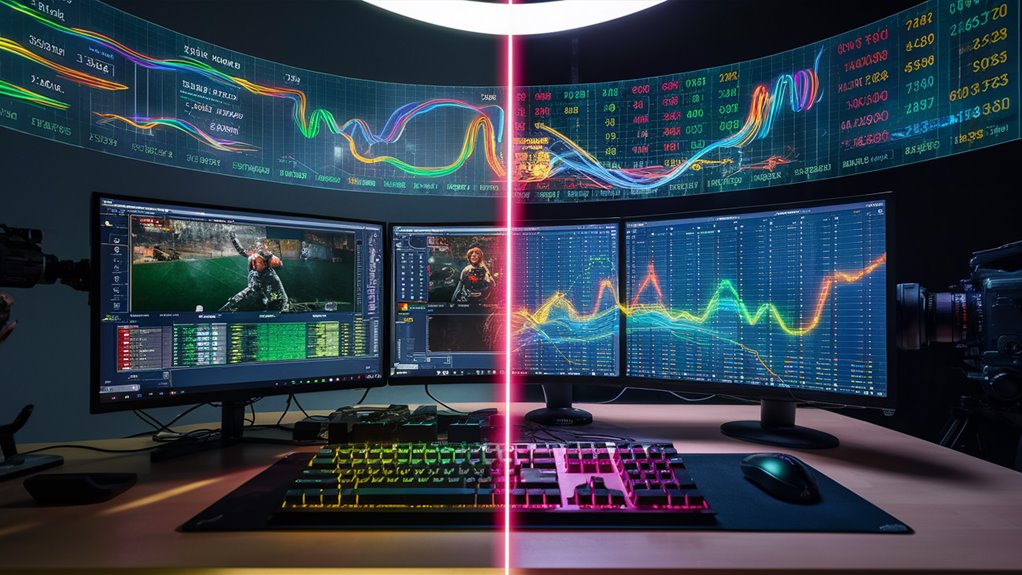
How Do Real-Time Analytics Work in Esports Matches?
Key Takeaways
- Real-time analytics platforms process thousands of data points per second during live matches
- Computer vision technology converts visual game data into actionable insights
- Key performance metrics include K/D ratios, resource management, and positioning patterns
Understanding Real-Time Data Processing
Real-time analytics platforms in esports process thousands of data points each second to generate instant insights during matches.
These sophisticated systems track multiple game elements simultaneously, including player positions, weapon usage, resource management, and team formations.
Every in-game action contributes to continuously updated win probability calculations.
Advanced Computer Vision Analysis
Modern analytics platforms leverage computer vision technology to transform game streams into structured data. For MOBA games like League of Legends or Dota 2, the system monitors:
- Objective control patterns
- Gold differences between teams
- Team fight outcomes and effectiveness
- Map movement and positioning
In FPS games like CS:GO, critical tracking elements include:
- Economy management decisions
- Map control percentages
- Player accuracy statistics
- Round win conditions
Performance Metrics and Prediction Models
The most reliable predictive insights come from analyzing multiple data points:
- Kill/death ratios
- Resource efficiency scores
- Positioning patterns
- Historical performance against specific opponents
- Team strategy adaptation rates
Risk Assessment and Management
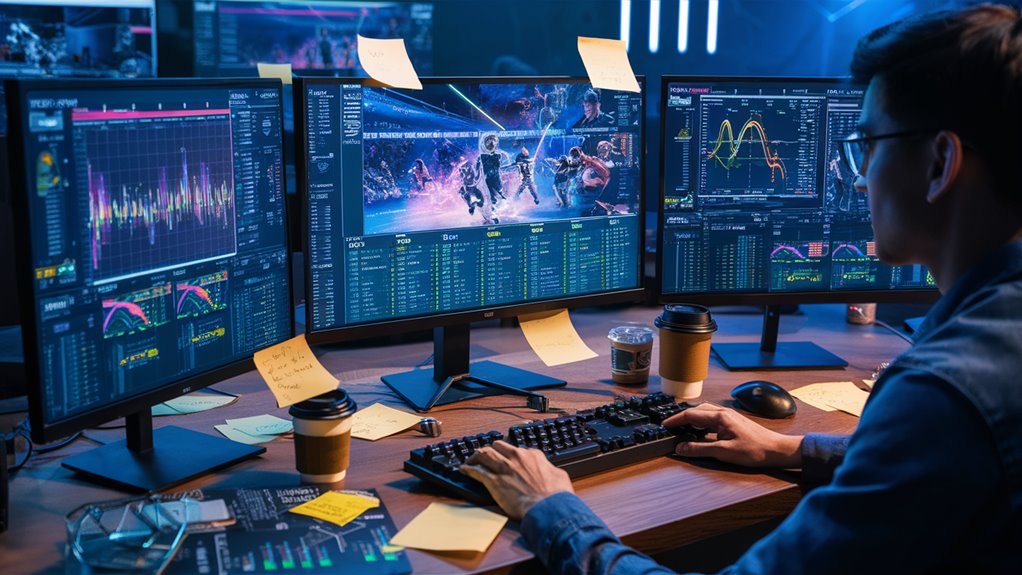
How Can You Effectively Assess and Manage Risks in Esports Betting?
Key Takeaways:
- Systematic risk assessment combines quantitative metrics and qualitative analysis
- Diversification across games and tournaments reduces single-event exposure
- Regular monitoring of betting patterns and market indicators prevents major losses
- Automated alerts and risk scoring systems enhance detection of suspicious activities
Understanding Risk Assessment Fundamentals
Effective risk assessment in esports betting requires monitoring key indicators, including betting patterns, player performance variations, and market volatility.
A systematic approach helps identify potential risks and implement appropriate mitigation strategies before they impact your betting outcomes.
Establishing Clear Risk Parameters
Set specific risk thresholds and betting limits based on:
- Historical win-loss ratios
- Odds fluctuation patterns
- Potential manipulation indicators
- Maximum exposure limits per event
Implementing Detection Systems
Deploy automated monitoring systems to track:
- Suspicious betting activities
- Unusual player behavior
- Sudden odds 먹튀검증 커뮤니티 movements
- Market irregularities
Portfolio Diversification Strategies
Minimize risk exposure through:
- Multi-game betting distribution
- Tournament-level diversification
- Varied bet type allocation
- Strategic stake sizing
Risk Scoring Framework
Develop a comprehensive scoring system incorporating:
- Team stability metrics
- Player roster changes
- Tournament significance levels
- Historical performance data
- Market condition indicators
Regular updates to risk models ensure continued effectiveness as market conditions evolve.
Combining quantitative data with qualitative assessments of team dynamics and competitive environments creates a robust risk evaluation framework for informed decision-making.
Future of Predictive Betting Systems
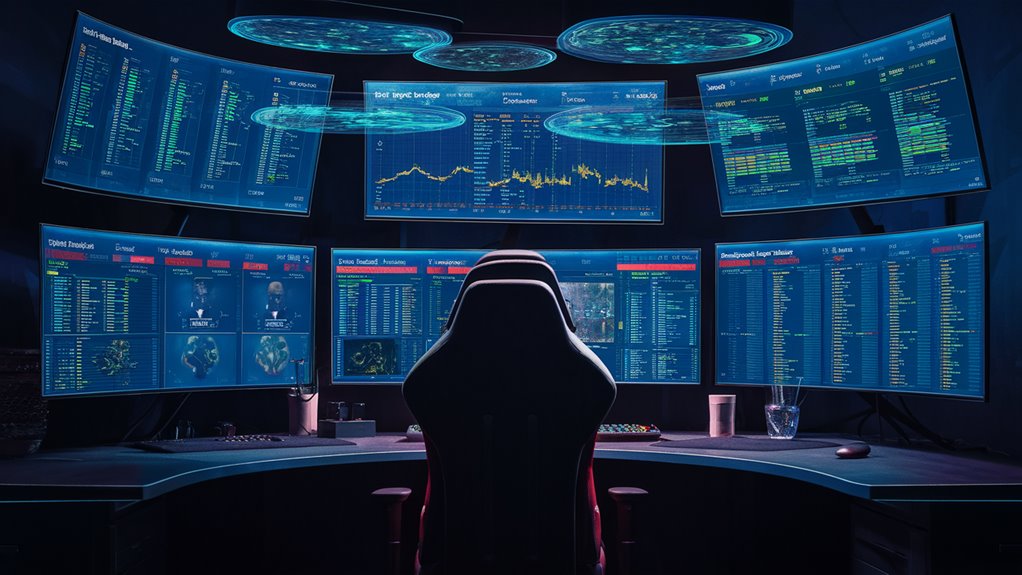
What’s the Future of AI-Powered Predictive Betting in Esports?
Key Takeaways
- AI and machine learning systems are revolutionizing esports betting predictions
- Blockchain technology enables secure, transparent betting transactions
- Virtual and augmented reality create immersive betting experiences
- Quantum computing promises unprecedented prediction accuracy
How AI Is Transforming Betting Predictions
Predictive betting systems in esports are undergoing rapid transformation through artificial intelligence and machine learning technologies.
These systems process real-time data from multiple sources:
- Player 개인 도박보너스 받는법 performance metrics
- Team dynamics analysis
- Meta-game shift patterns
- Social media sentiment
- Team announcements
- Player communications
Blockchain’s Role in Secure Betting
Blockchain technology delivers two critical improvements to betting systems:
- Smart contracts that automate payment processing
- Distributed ledgers that enhance transparency
- Fraud reduction through immutable records
Immersive Betting Experiences
Virtual and augmented reality technologies are creating new ways for users to interact with betting systems:
- 3D visualization of betting data
- Interactive pattern recognition
- Real-time analytics displays
- Mobile accessibility for instant betting
The Quantum Computing advantage
Advanced computing capabilities are set to revolutionize predictive accuracy through:
- Processing vast historical match datasets
- Complex pattern recognition
- Real-time adjustment of odds
- Advanced risk assessment modeling
These systems continually evolve to accommodate new games and changing gameplay mechanics, requiring minimal manual intervention while maintaining high accuracy in predictions.