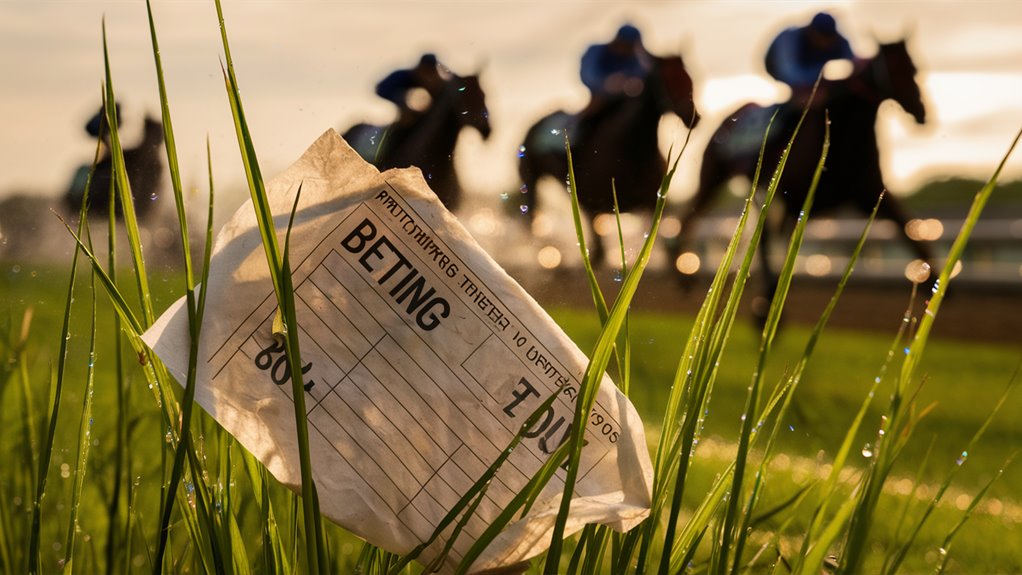
Hidden Verge Bets: Mastering Undervalued Market Opportunities
Understanding Hidden Verge Trading Dynamics
Hidden verge bets emerge at the critical intersection of market psychology and statistical inefficiencies, where undervalued opportunities remain concealed from mainstream traders. These overlooked positions represent significant profit potential when accurately identified through advanced analytical frameworks.
Data-Driven Analysis and Pattern Recognition
Statistical analysis combined with multi-timeframe pattern recognition forms the foundation for identifying premium trading opportunities. By leveraging machine learning algorithms to process extensive market datasets, traders can uncover hidden correlations and behavioral patterns that signal potential market mispricing.
Advanced Market Analysis Techniques
- Volatility ratio analysis
- Correlation dynamics monitoring
- Market sentiment indicators
- Statistical arbitrage signals
- Behavioral pattern recognition
Risk Management and Position Building
Implementing systematic risk controls and strategic position building ensures optimal exploitation of boundary opportunities. Position sizing, risk-adjusted returns, and correlation-based hedging create a robust framework for capitalizing on hidden verge scenarios.
#
Frequently Asked Questions
Q: What are hidden verge bets?
A: Hidden verge bets are undervalued market opportunities that exist due to psychological biases and statistical inefficiencies in market pricing.
Q: How can traders identify hidden verge opportunities?
A: Traders can identify these opportunities through data-driven analysis, machine learning algorithms, and multi-timeframe pattern recognition.
Q: What role does market psychology play?
A: Market psychology creates behavioral patterns that lead to mispricing, generating opportunities for informed traders to capitalize on collective market inefficiencies.
Q: Why are risk management systems crucial?
A: Risk management systems protect capital while maximizing returns through systematic position sizing and correlation-based hedging strategies.
Q: How do machine learning algorithms enhance trading decisions?
A: Machine learning algorithms process vast amounts of market data to identify patterns and correlations that human analysts might miss, leading to more accurate trading decisions.
Understanding Verge Bet Psychology
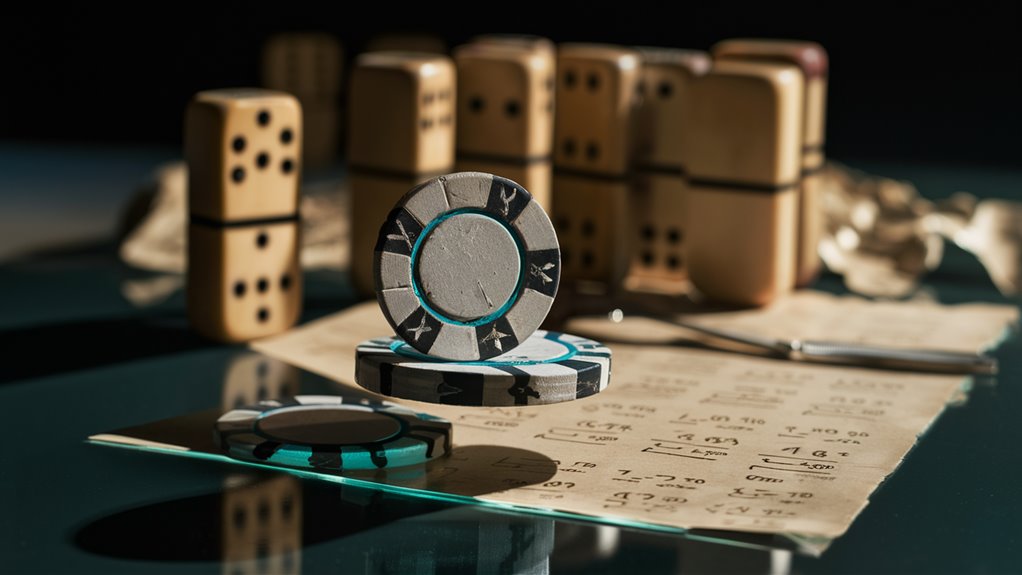
Understanding Verge Bet Psychology: A Comprehensive Guide
The Psychology Behind Verge Betting
Verge betting psychology operates at the intersection of risk assessment and market timing.
Successful verge betting requires deep understanding of both market inefficiencies and cognitive biases that create profitable opportunities. Many traders overlook these edges due to anchoring bias and risk aversion behaviors.
Identifying Verge Zones and Market Opportunities
Verge zones emerge in transitional states between established price levels, representing areas where probability assessments become particularly unreliable.
These high-value opportunities typically manifest during periods of low market liquidity or when markets process new information.
Traders who recognize these patterns can capitalize on systematic pricing inefficiencies.
Key Psychological Components for Success
Optimal verge betting requires three critical psychological attributes:
- Comfort with ambiguity
- Resistance to herd mentality
- Advanced pattern recognition skills
Advanced Strategy Implementation
Successful traders exploit the market’s tendency to underestimate state changes near established boundaries.
By focusing on these psychological blind spots, investors can identify opportunities where collective market psychology creates valuable pricing inefficiencies at critical transition points.
#
Frequently Asked Questions
Q: What’re verge zones in betting psychology?
A: Verge zones are transitional areas between established price levels where probability assessments become less reliable and present unique opportunities.
Q: How do cognitive biases affect verge betting?
A: Cognitive biases, particularly anchoring bias and herd mentality, create market inefficiencies that experienced traders can exploit.
Q: What psychological skills are essential for verge betting?
A: Key psychological skills include comfort with ambiguity, resistance to herd behavior, and rapid pattern recognition.
Q: When do verge betting opportunities typically occur?
A: These opportunities most commonly emerge during periods of low liquidity or when markets are processing new information.
Q: How can traders identify psychological blind spots in markets?
A: Traders can identify blind spots by monitoring collective market behavior and recognizing when traditional probability assessments become unreliable.
Identifying Statistical Market Inefficiencies
Identifying Statistical Market Inefficiencies: A Comprehensive Guide
Understanding Market Inefficiency Patterns
Statistical market inefficiencies create valuable opportunities for strategic trading through predictable patterns and systematic analysis. These inefficiencies typically emerge during high-volatility periods when emotional trading dominates rational decision-making, leading to exploitable market conditions.
Key Statistical Indicators
Standard deviation analysis against historical volatility provides crucial insights into market mispricing. Three primary inefficiency categories emerge:
- Overnight Gap Mispricing
- Correlation Breakdown between related assets
- Momentum Overshoots in trending markets
Advanced Statistical Analysis Methods
Quantitative indicators measuring market sentiment versus actual price movements reveal profitable opportunities. The most significant inefficiencies occur when implied volatility diverges from realized volatility, creating exploitable gaps across multiple timeframes.
Common Market Inefficiency Manifestations:
- Mispriced Options
- Overextended Futures Contracts
- Temporary Arbitrage Opportunities
## Frequently Asked Questions
Q: What’re the primary indicators of market inefficiency?
A: Key indicators include divergence between 토토검증사이트 implied and realized volatility, correlation breakdowns, and systematic price pattern deviations.
Q: How do volatility metrics help identify trading opportunities?
A: Volatility metrics reveal discrepancies between market pricing and statistical norms, highlighting potential profit opportunities.
Q: When do market inefficiencies typically occur?
A: Inefficiencies commonly emerge during high-volatility periods and when emotional trading overshadows rational price discovery.
Q: What tools are essential for identifying statistical inefficiencies?
A: Standard deviation analysis tools, correlation metrics, and volatility tracking systems are crucial for identifying inefficiencies.
Q: How can traders capitalize on identified inefficiencies?
A: Traders can exploit inefficiencies through strategic position-taking in options, futures, and correlated instrument arbitrage.
Risk Assessment Methods
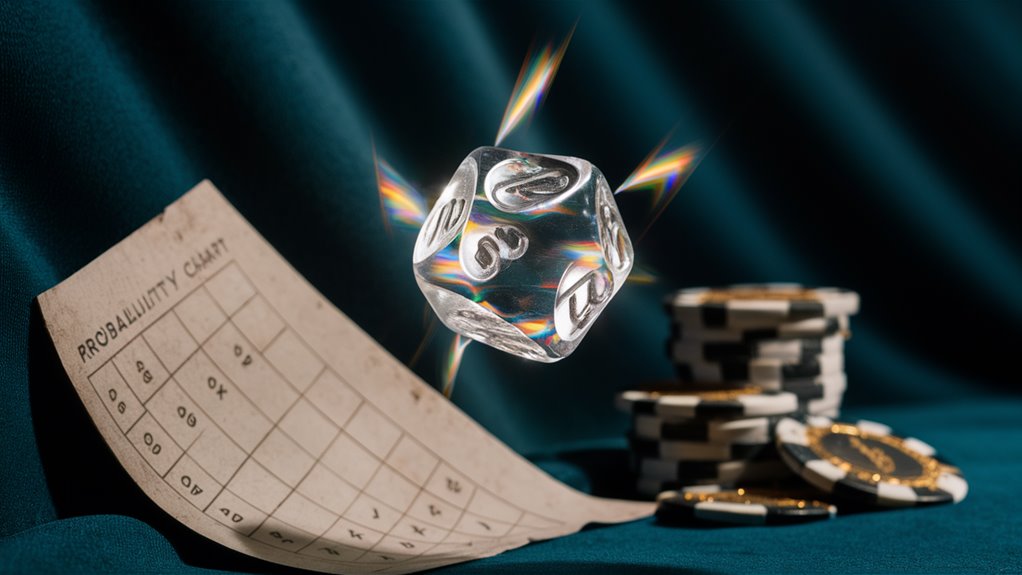
Comprehensive Guide to Risk Assessment Methods
Core Components of Risk Analysis
Risk assessment forms the foundation of modern portfolio management, enabling investors to identify market inefficiencies while maintaining stability.
A multi-layered approach to risk evaluation helps detect potential downsides before they materialize.
Through systematic analysis of historical volatility patterns, correlation matrices, and maximum drawdown scenarios, investors can effectively quantify risk-adjusted return potential.
Three Essential Risk Categories
Market Risk (Beta)
Value at Risk (VaR) calculations at 95% and 99% confidence intervals provide crucial insights into potential portfolio losses.
This systematic approach helps measure exposure to broader market movements and economic factors.
Specific Risk (Idiosyncratic Factors)
Deep fundamental analysis encompasses company-specific factors, industry dynamics, and regulatory impacts.
This granular assessment reveals unique risks affecting individual securities and sectors.
Operational Risk (Execution)
Monitoring liquidity constraints, position sizing, and counterparty exposure ensures smooth portfolio management.
These factors directly impact trading efficiency and overall performance.
Advanced Risk Management Framework
Stress testing under various market conditions provides essential portfolio resilience data.
A proprietary scoring system weights risk factors based on current market dynamics.
Position limits and automated stop-loss triggers protect against excessive exposure to single positions.
Frequently Asked Questions
- What’s Value at Risk (VaR)?
- How do you implement effective position sizing?
- Why is liquidity analysis important in risk assessment?
- How often should risk assessments be updated?
- What role do correlation matrices play in risk management?
Risk Management Best Practices
- Regular monitoring of risk metrics
- Dynamic adjustment of position sizes
- Continuous evaluation of market conditions
- Implementation of automated risk controls
- Regular stress testing and scenario analysis
Data-Driven Selection Strategies
Data-Driven Selection Strategies: A Comprehensive Guide
Understanding Modern Selection Analytics
Data-driven selection strategies leverage sophisticated algorithmic analysis and machine learning models to identify high-probability trading opportunities. The combination of historical price data, market sentiment indicators, and real-time analytics creates a robust framework for detecting valuable market positions.
Advanced pattern analysis across multiple timeframes enables the identification of market inefficiencies and hidden opportunities.
Core Components of Data Selection
Statistical Analysis Framework
Statistical arbitrage signals form the foundation of effective data-driven selection. These mathematical indicators help quantify market movements and identify potential price discrepancies.
The systematic approach eliminates emotional bias while maximizing analytical precision.
Volatility Assessment
Volatility pattern analysis provides crucial insights into market behavior and risk levels. Advanced algorithms process market fluctuations to detect trading opportunities and potential market shifts.
This analytical layer helps establish more accurate price movement predictions.
Correlation Dynamics
Correlation matrices reveal interconnected market relationships and potential divergences. By mapping these relationships, traders can identify risk-adjusted opportunities and validate trading decisions through multiple data points.
Advanced Implementation Strategies
The integration of machine learning algorithms with traditional market analysis creates a powerful selection framework.
This hybrid approach processes vast quantities of market data while maintaining alignment with established trading principles. The result is a systematic selection process that combines technological innovation with proven market wisdom.
Frequently Asked Questions
- What are the key components of data-driven selection?
- Statistical arbitrage analysis
- Volatility pattern recognition
- Correlation matrix mapping
- Machine learning integration
- Real-time market analytics
- How does machine learning enhance selection strategies?
- Processes large data sets efficiently
- Identifies complex market patterns
- Reduces human bias in analysis
- Enables real-time decision making
- Improves prediction accuracy
- What role do correlation matrices play?
- Map market relationships
- Identify trading opportunities
- Validate investment decisions
- Monitor risk exposure
- Track market inefficiencies
- How is market sentiment incorporated?
- Analysis of social media trends
- News sentiment tracking
- Investor behavior patterns
- Market momentum indicators
- Sentiment signal processing
- What makes a selection strategy effective?
- Systematic approach implementation
- Multiple data source integration
- Real-time analysis capabilities
- Risk management framework
- Continuous strategy optimization
#
Building Long-Term Winning Positions
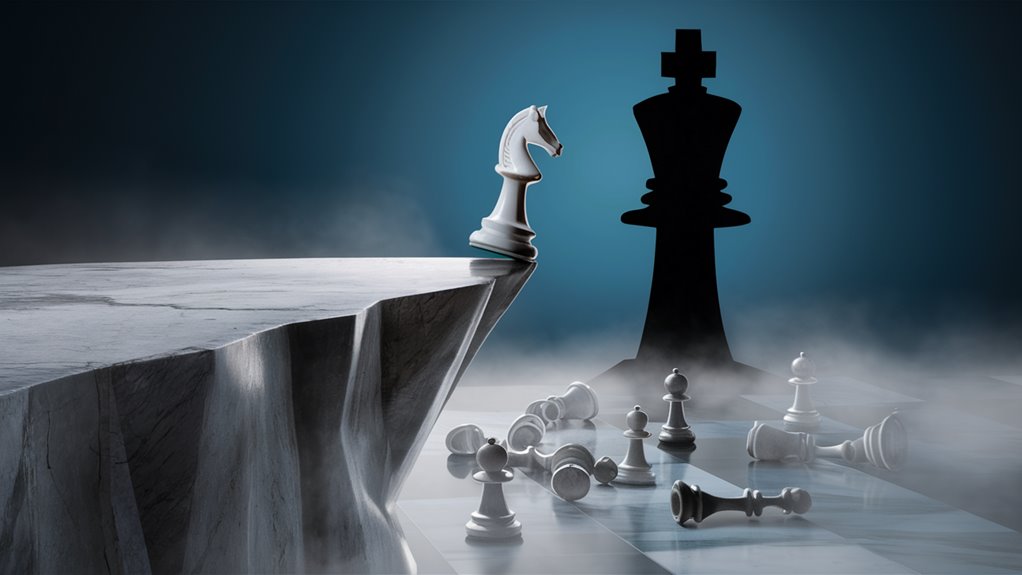
# Building Long-Term Winning Positions
Strategic Position Building Fundamentals
Success in establishing long-term winning positions requires the systematic integration of multiple data streams and disciplined execution strategies.
Combining historical price patterns, market sentiment indicators, and real-time flow analysis creates a robust framework for identifying high-probability opportunities.
Systematic tracking of these metrics reveals valuable market divergences and potential entry points.
Core Components for Sustainable Success
Position Management
Optimal position sizing relative to total capital forms the cornerstone of sustainable trading.
Dynamic risk adjustment based on market volatility helps maintain consistent exposure levels while protecting capital during turbulent periods.
Multi-Timeframe Analysis
Correlation analysis across multiple timeframes provides crucial insights into market relationships.
Inter-market analysis prevents overconcentration in correlated positions and enhances portfolio diversification.
Advanced Position Building Techniques
Methodical position accumulation using 15-20% initial allocation allows for strategic scaling.
This approach proves particularly effective in markets with structural inefficiencies.
Monitoring the realized-to-implied volatility ratio identifies optimal entry points where market pricing mechanisms temporarily misalign.
## Frequently Asked Questions
Q: What’re the key indicators for building winning positions?
A: Essential indicators include historical price patterns, market sentiment metrics, and real-time flow analysis.
Q: How should position sizing be determined?
A: Position sizing should be based on total capital, market volatility, and correlation analysis across different timeframes.
Q: What’s the optimal initial position size?
A: Start with 15-20% of intended position size, allowing room for strategic scaling as market conditions confirm the trading thesis.
Q: How can traders identify market inefficiencies?
A: Monitor the realized-to-implied volatility ratio and track inter-market relationships to spot pricing discrepancies.
Q: What role does correlation analysis play?
A: Correlation analysis helps prevent overconcentration in similar positions and ensures proper portfolio diversification across markets.